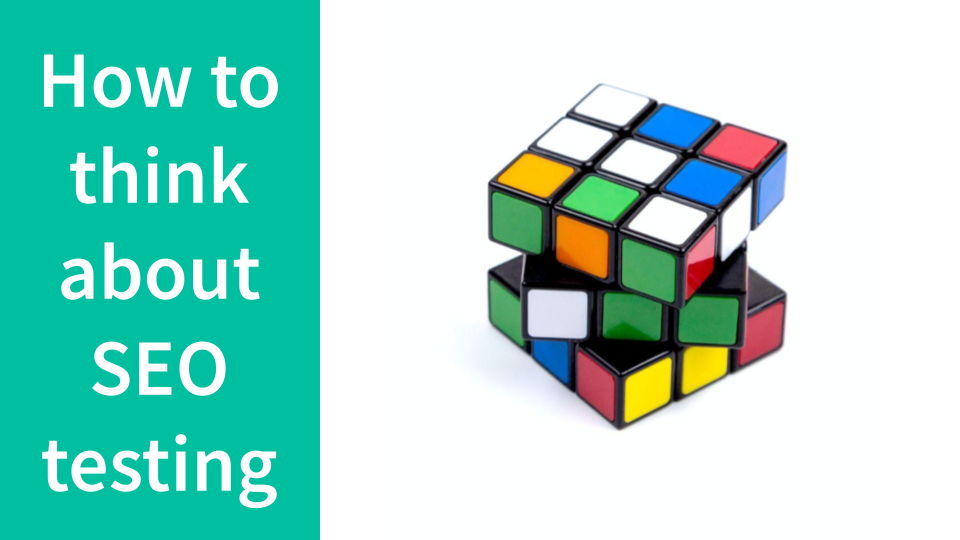
Many businesses these days don’t see their customers face to face and instead serve them (sometimes millions of them) on their websites. With the shift to online, the idea of being customer centric isn’t fading. In fact, most customers expect businesses to understand their needs. But how can sites that serve millions of visitors a year understand their customers' needs when interacting with their website?
Many companies look to SEO testing as one answer. But in doing so, some get caught up in the testing variables, and the results' significance, forgetting about the objective behind the test in the first place—to improve customer experience and increase revenue. Instead of getting caught up in how to handle non-statistically significant results, we have a simple system for how to approach SEO split testing results.
Learn more about our approach to SEO split-testing, why it is beneficial even if you don’t have statistical significance, and the ways we work with our customers to run and analyse tests.
- What to do when you don’t reach statistical significance
- When to deploy a test
- When to not deploy
- Weighing the potential downsides to deploying non-statistically significant findings
What to do when you don’t reach statistical significance
When a test is based on a strong hypothesis, and our analysis is failing to reach statistical significance for our chosen level of confidence, our team makes a consulting-style call on whether to recommend that the customer deploys the change to their whole site / section.
This is the framework that our team uses in these scenarios. It is based on the strength of the original hypothesis and how expensive it is to make and maintain the change:
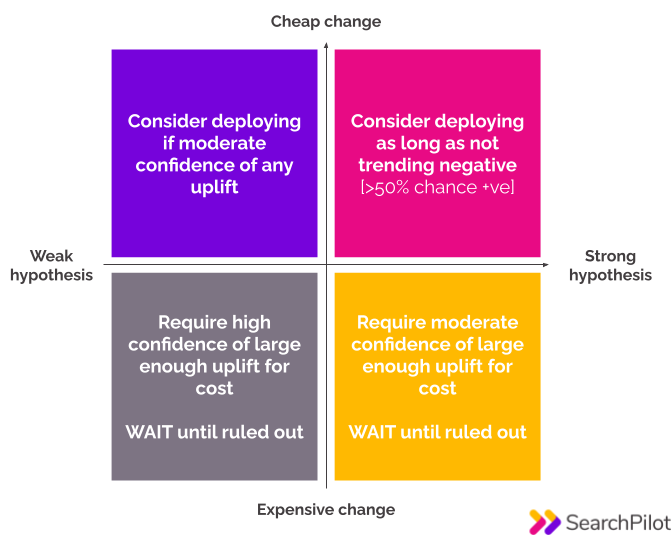
When to deploy a test
We are here to help our customers capture any competitive benefit available, whether we have proved a statistically-significant result or not, so we default to deploy in these two scenarios:
- Capturing uplifts smaller than we can measure - We know that there are uplifts that are too small for us to detect with statistical confidence - both in theory and in practice. There is a world of marginal gains that are smaller than this that can add up to a competitive advantage if we can on average deploy more small positive changes than negative ones.
- Align with future algorithm updates - When we build hypotheses from our understanding of theoretical information retrieval, or from user preferences that we believe Google might move their algorithms toward in the future, we may run tests that are not immediately positive, but that have a positive expected future return.
In either case, we will want to ensure that our testing has indicated that we don’t have any reason to suspect the change will have a negative impact. In general this just means that it mustn’t be trending negative, which means it has a 50+% chance of being positive.
Pink quadrant - strong hypothesis / cheap change
When you have a strong hypothesis and it is an easy change to make and maintain, then we can recommend deploying. The chances of a positive outcome will outweigh the potential risks over time.
Purple quadrant - weak hypothesis / cheap change
The same philosophy also applies if it is cheap to reverse the decision and you have a weaker hypothesis. In this case we want to see at least some statistical indication of a positive impact - typically a positive result at a lower statistical significance. Again, when you weigh the potential upside vs the risks, the upside wins out over time.
In both of these scenarios, we’re taking a pragmatic approach to roll out the changes. Here is why.
If we only allow ourselves to deploy changes that are provably beneficial already, we may lose out to competitors who are prepared to act on speculation or announcements of what Google might value more highly in the future.
When not to deploy
In the bottom half of the quadrant, we might recommend not deploying the change we tested for any of four reasons:
- The test has shown with statistical confidence that the change will have a negative impact
- While not reaching statistical significance, the data shows it is more likely damaging than helping, or hasn’t provided enough evidence of an uplift when considering a test with a relatively weak hypothesis
- The scale of the potential uplift is too small to justify the cost / maintenance of this feature
- It is a risky or costly change and the range of uncertainty is simply too wide to make a call to deploy based on the data
Orange quadrant - strong hypothesis / expensive change
The decision on whether or not to deploy gets trickier when the changes are more costly or harder to maintain. When this is the case we don’t recommend deploying without reasonably strong evidence of an uplift, because of the potential risks.
Grey quadrant - weak hypothesis / expensive change
When you have a weak hypothesis and it’s expensive we don’t recommend deploying without very strong evidence of an uplift. We only recommend deploying this kind of change when we reach true statistical significance.
Weighing the potential downsides to deploying non-statistically significant findings
Beyond the statistical risks (most obviously, the risk of deploying the occasional change that is actually negative), the biggest strategic challenge to this approach is that when we look back at a sequence of tests and enhancements, we won’t have strong numerical arguments for all of them to compare to their implementation cost.
It’s worth remembering that statistical confidence is worth something in the reviews / retrospectives that look at uplifts generated by the activity. We may find some customers push more towards waiting longer for statistical confidence and / or tweaking and re-running tests in search of stronger uplifts because they particularly value this confidence. This is fine, but our default approach is to seek the greatest business benefit for customers rather than the greatest certainty.
One of the other risks with this approach is the chance that serious experimentation teams and individuals with a scientific bent may feel that we are being misleading or dishonest. For instance, any language like “nearly significant” or “trending to significance” is widely derided and mocked.
When a test hasn’t reached statistical significance, then at worst we should act as if the data is noise rather than the temptation to assume it is evidence that the test has failed. In other words, you can use the strength of the hypothesis to make the case that you should deploy anyway, but without using the lower performance thresholds to make the case for additional tests in the same area or for developing new features based on the test.
In sum, going back to our decision framework, when in the top half of the quadrant, we weigh the benefits of quick decisions to deploy with the benefit of waiting for more data to get higher confidence or greater certainty of the magnitude of the uplift. The top half is about balancing probabilities, avoiding drops, and winning in the long run, while the bottom half is about justifying the cost of the change and getting ROI data.
In the long run, we aim to be successful by giving our customers a genuine competitive advantage. At the margin, if we have to choose between being certain something brings an uplift, or capturing any uplift that is there, we should choose the latter, especially when we believe there is only a small chance of a negative impact.
Image credit: Volodymyr Hryshchenko.